ノート
完全なサンプルコードをダウンロードするには、ここをクリックしてください
注釈付きヒートマップの作成#
2 つの独立変数に依存するデータを、色分けされたイメージ プロットとして表示することが望ましい場合がよくあります。これはしばしばヒートマップと呼ばれます。データがカテゴリの場合、これはカテゴリ ヒートマップと呼ばれます。
Matplotlib のimshow
機能により、このようなプロットの作成が特に簡単になります。
次の例は、注釈を使用してヒートマップを作成する方法を示しています。簡単な例から始めて、それを拡張して汎用関数として使用できるようにします。
単純なカテゴリ ヒートマップ#
いくつかのデータを定義することから始めます。必要なのは、データを色分けして定義する 2D リストまたは配列です。次に、カテゴリの 2 つのリストまたは配列も必要です。もちろん、これらのリストの要素の数は、それぞれの軸に沿ったデータと一致する必要があります。ヒートマップ自体はimshow
、ラベルがカテゴリに設定されたプロットです。set_xticks
目盛りの位置 ( ) と目盛りラベル ( )の両方を設定することが重要であることに注意してくださいset_xticklabels
。そうしないと、それらが同期しなくなります。位置は昇順の整数であり、目盛りラベルは表示するラベルです。Text
最後に、各セル内にそのセルの値を示す を作成することで、データ自体にラベルを付けることができます。
import numpy as np
import matplotlib
import matplotlib as mpl
import matplotlib.pyplot as plt
vegetables = ["cucumber", "tomato", "lettuce", "asparagus",
"potato", "wheat", "barley"]
farmers = ["Farmer Joe", "Upland Bros.", "Smith Gardening",
"Agrifun", "Organiculture", "BioGoods Ltd.", "Cornylee Corp."]
harvest = np.array([[0.8, 2.4, 2.5, 3.9, 0.0, 4.0, 0.0],
[2.4, 0.0, 4.0, 1.0, 2.7, 0.0, 0.0],
[1.1, 2.4, 0.8, 4.3, 1.9, 4.4, 0.0],
[0.6, 0.0, 0.3, 0.0, 3.1, 0.0, 0.0],
[0.7, 1.7, 0.6, 2.6, 2.2, 6.2, 0.0],
[1.3, 1.2, 0.0, 0.0, 0.0, 3.2, 5.1],
[0.1, 2.0, 0.0, 1.4, 0.0, 1.9, 6.3]])
fig, ax = plt.subplots()
im = ax.imshow(harvest)
# Show all ticks and label them with the respective list entries
ax.set_xticks(np.arange(len(farmers)), labels=farmers)
ax.set_yticks(np.arange(len(vegetables)), labels=vegetables)
# Rotate the tick labels and set their alignment.
plt.setp(ax.get_xticklabels(), rotation=45, ha="right",
rotation_mode="anchor")
# Loop over data dimensions and create text annotations.
for i in range(len(vegetables)):
for j in range(len(farmers)):
text = ax.text(j, i, harvest[i, j],
ha="center", va="center", color="w")
ax.set_title("Harvest of local farmers (in tons/year)")
fig.tight_layout()
plt.show()

ヘルパー関数コード スタイルの使用#
コーディング スタイルで説明したように、その ようなコードを再利用して、さまざまな入力データおよび/またはさまざまな軸で何らかのヒートマップを作成することができます。入力としてデータと行と列のラベルを取り、プロットをカスタマイズするために使用される引数を許可する関数を作成します
ここでは、上記に加えて、カラーバーを作成し、ラベルをヒートマップの下ではなく上に配置します。注釈は、ピクセルの色とのコントラストを向上させるために、しきい値に応じて異なる色を取得します。最後に、周囲の軸のスパインをオフにし、白い線のグリッドを作成してセルを分離します。
def heatmap(data, row_labels, col_labels, ax=None,
cbar_kw=None, cbarlabel="", **kwargs):
"""
Create a heatmap from a numpy array and two lists of labels.
Parameters
----------
data
A 2D numpy array of shape (M, N).
row_labels
A list or array of length M with the labels for the rows.
col_labels
A list or array of length N with the labels for the columns.
ax
A `matplotlib.axes.Axes` instance to which the heatmap is plotted. If
not provided, use current axes or create a new one. Optional.
cbar_kw
A dictionary with arguments to `matplotlib.Figure.colorbar`. Optional.
cbarlabel
The label for the colorbar. Optional.
**kwargs
All other arguments are forwarded to `imshow`.
"""
if ax is None:
ax = plt.gca()
if cbar_kw is None:
cbar_kw = {}
# Plot the heatmap
im = ax.imshow(data, **kwargs)
# Create colorbar
cbar = ax.figure.colorbar(im, ax=ax, **cbar_kw)
cbar.ax.set_ylabel(cbarlabel, rotation=-90, va="bottom")
# Show all ticks and label them with the respective list entries.
ax.set_xticks(np.arange(data.shape[1]), labels=col_labels)
ax.set_yticks(np.arange(data.shape[0]), labels=row_labels)
# Let the horizontal axes labeling appear on top.
ax.tick_params(top=True, bottom=False,
labeltop=True, labelbottom=False)
# Rotate the tick labels and set their alignment.
plt.setp(ax.get_xticklabels(), rotation=-30, ha="right",
rotation_mode="anchor")
# Turn spines off and create white grid.
ax.spines[:].set_visible(False)
ax.set_xticks(np.arange(data.shape[1]+1)-.5, minor=True)
ax.set_yticks(np.arange(data.shape[0]+1)-.5, minor=True)
ax.grid(which="minor", color="w", linestyle='-', linewidth=3)
ax.tick_params(which="minor", bottom=False, left=False)
return im, cbar
def annotate_heatmap(im, data=None, valfmt="{x:.2f}",
textcolors=("black", "white"),
threshold=None, **textkw):
"""
A function to annotate a heatmap.
Parameters
----------
im
The AxesImage to be labeled.
data
Data used to annotate. If None, the image's data is used. Optional.
valfmt
The format of the annotations inside the heatmap. This should either
use the string format method, e.g. "$ {x:.2f}", or be a
`matplotlib.ticker.Formatter`. Optional.
textcolors
A pair of colors. The first is used for values below a threshold,
the second for those above. Optional.
threshold
Value in data units according to which the colors from textcolors are
applied. If None (the default) uses the middle of the colormap as
separation. Optional.
**kwargs
All other arguments are forwarded to each call to `text` used to create
the text labels.
"""
if not isinstance(data, (list, np.ndarray)):
data = im.get_array()
# Normalize the threshold to the images color range.
if threshold is not None:
threshold = im.norm(threshold)
else:
threshold = im.norm(data.max())/2.
# Set default alignment to center, but allow it to be
# overwritten by textkw.
kw = dict(horizontalalignment="center",
verticalalignment="center")
kw.update(textkw)
# Get the formatter in case a string is supplied
if isinstance(valfmt, str):
valfmt = matplotlib.ticker.StrMethodFormatter(valfmt)
# Loop over the data and create a `Text` for each "pixel".
# Change the text's color depending on the data.
texts = []
for i in range(data.shape[0]):
for j in range(data.shape[1]):
kw.update(color=textcolors[int(im.norm(data[i, j]) > threshold)])
text = im.axes.text(j, i, valfmt(data[i, j], None), **kw)
texts.append(text)
return texts
上記により、実際のプロット作成をかなりコンパクトに保つことができます。
fig, ax = plt.subplots()
im, cbar = heatmap(harvest, vegetables, farmers, ax=ax,
cmap="YlGn", cbarlabel="harvest [t/year]")
texts = annotate_heatmap(im, valfmt="{x:.1f} t")
fig.tight_layout()
plt.show()

より複雑なヒートマップの例#
以下では、さまざまなケースに適用し、さまざまな引数を使用して、以前に作成した関数の汎用性を示します。
np.random.seed(19680801)
fig, ((ax, ax2), (ax3, ax4)) = plt.subplots(2, 2, figsize=(8, 6))
# Replicate the above example with a different font size and colormap.
im, _ = heatmap(harvest, vegetables, farmers, ax=ax,
cmap="Wistia", cbarlabel="harvest [t/year]")
annotate_heatmap(im, valfmt="{x:.1f}", size=7)
# Create some new data, give further arguments to imshow (vmin),
# use an integer format on the annotations and provide some colors.
data = np.random.randint(2, 100, size=(7, 7))
y = ["Book {}".format(i) for i in range(1, 8)]
x = ["Store {}".format(i) for i in list("ABCDEFG")]
im, _ = heatmap(data, y, x, ax=ax2, vmin=0,
cmap="magma_r", cbarlabel="weekly sold copies")
annotate_heatmap(im, valfmt="{x:d}", size=7, threshold=20,
textcolors=("red", "white"))
# Sometimes even the data itself is categorical. Here we use a
# `matplotlib.colors.BoundaryNorm` to get the data into classes
# and use this to colorize the plot, but also to obtain the class
# labels from an array of classes.
data = np.random.randn(6, 6)
y = ["Prod. {}".format(i) for i in range(10, 70, 10)]
x = ["Cycle {}".format(i) for i in range(1, 7)]
qrates = list("ABCDEFG")
norm = matplotlib.colors.BoundaryNorm(np.linspace(-3.5, 3.5, 8), 7)
fmt = matplotlib.ticker.FuncFormatter(lambda x, pos: qrates[::-1][norm(x)])
im, _ = heatmap(data, y, x, ax=ax3,
cmap=mpl.colormaps["PiYG"].resampled(7), norm=norm,
cbar_kw=dict(ticks=np.arange(-3, 4), format=fmt),
cbarlabel="Quality Rating")
annotate_heatmap(im, valfmt=fmt, size=9, fontweight="bold", threshold=-1,
textcolors=("red", "black"))
# We can nicely plot a correlation matrix. Since this is bound by -1 and 1,
# we use those as vmin and vmax. We may also remove leading zeros and hide
# the diagonal elements (which are all 1) by using a
# `matplotlib.ticker.FuncFormatter`.
corr_matrix = np.corrcoef(harvest)
im, _ = heatmap(corr_matrix, vegetables, vegetables, ax=ax4,
cmap="PuOr", vmin=-1, vmax=1,
cbarlabel="correlation coeff.")
def func(x, pos):
return "{:.2f}".format(x).replace("0.", ".").replace("1.00", "")
annotate_heatmap(im, valfmt=matplotlib.ticker.FuncFormatter(func), size=7)
plt.tight_layout()
plt.show()
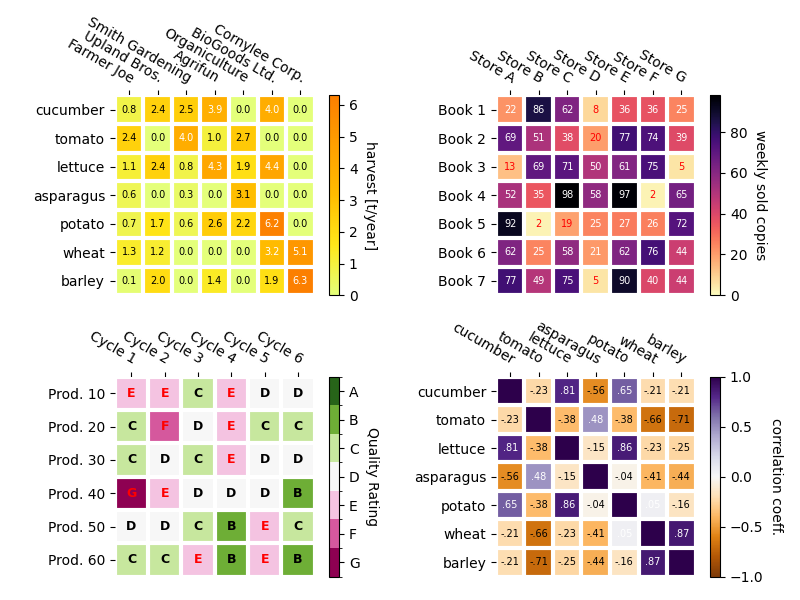
参考文献
この例では、次の関数、メソッド、クラス、およびモジュールの使用が示されています。
スクリプトの合計実行時間: ( 0 分 2.652 秒)